In today’s interconnected world, where the internet plays a vital role in every aspect of our lives, the importance of cybersecurity cannot be overstated. Today, cybercriminals are becoming increasingly sophisticated in their attacks, leveraging new technologies and techniques to breach security systems and steal sensitive information.
This state of affairs has required a paradigm shift in cybersecurity strategies for organizations. One such transformative force that has emerged in recent years is the use of Artificial Intelligence (AI) to bolster cybersecurity.
Contrary to popular opinion that AI has only exacerbated the risks of cybersecurity, it is now proving to be a powerful tool in countering security issues. Today, organizations are harnessing the capabilities of AI to analyze vast amounts of data to enhance their threat detection and response mechanisms.
According to a report by Capgemini, 61% of organizations that have implemented AI in their cybersecurity operations have seen a reduction in the time taken to detect and respond to breaches, and 56% have seen a reduction in the overall cost of cybersecurity.
Harnessing the Power of AI for Cybersecurity
According to the Cyber Security Intelligence Index Report published by IBM, human error was a major contributing cause in 95% of all breaches. AI brings a new level of efficiency, speed, and accuracy to the field of cybersecurity, empowering security teams to stay ahead of malicious actors. From threat detection and analysis to automated incident response, AI has revolutionized the way we protect our digital assets.
Here are five ways you can leverage AI in your cybersecurity initiatives.
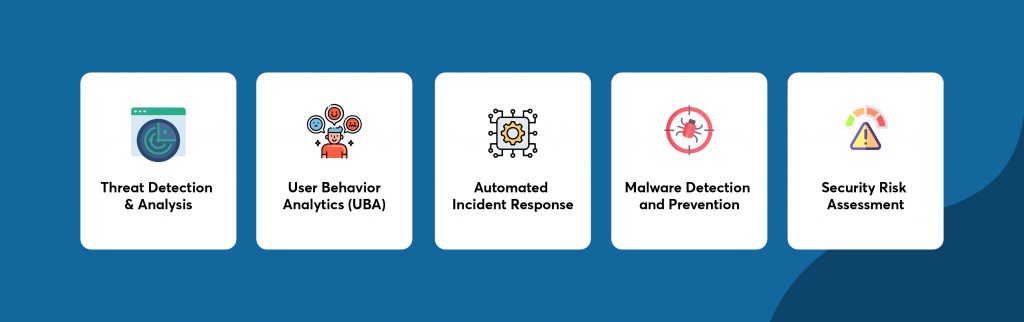
1. Threat Detection & Analysis
By leveraging advanced machine learning algorithms, AI systems can analyze vast amounts of data, detect patterns, and identify potential threats with remarkable accuracy. These systems can continuously monitor network traffic, endpoint activities, and user behavior to look for anomalies or potential security breaches. The reach of these systems is not just restricted to structured data. They can also analyze and categorize unstructured data, such as social media feeds and dark web forums, to detect emerging threats and malicious activities using natural language processing techniques.
A prominent example of using AI for threat detection and analysis is the use of Microsoft’s Cyber Signals. This system is being used by C-Level executives to analyze 24 trillion security signals, 40 nation-state groups, and 140 hacker groups to produce cyber threat intelligence. Imagine doing the same work using human staff!
2. User Behavior Analytics (UBA)
User Behavior Analytics (UBA) is the process of tracking, collecting, and assessing user data and activities using monitoring systems. These systems leverage AI to analyze and understand user behavior patterns across various digital platforms and detect deviations from normal behavior, such as unusual login locations, atypical data access patterns, or abnormal data transfer volumes.
As mentioned previously, UBA systems can’t take any action on their own. Instead, they can be configured to alert the security team. These systems can continuously learn and adapt to evolving user behavior, allowing for real-time detection and response to potential security breaches. By combining AI with UBA, organizations can proactively identify and mitigate risks, enhance threat prevention, and strengthen their overall cybersecurity posture.
3. Automated Incident Response
AI plays a crucial role in Automated Incident Response (AIR) by enabling organizations to quickly detect, analyze, and respond to cybersecurity incidents. Incident response systems powered by AI can autonomously analyze large volumes of security alerts, identify the severity of threats, and determine the appropriate response actions.
Through machine learning algorithms, these systems can continuously learn from past incidents and adapt their response strategies to improve efficiency and effectiveness. By automating incident response, AI-powered systems can help organizations minimize response time, reduce manual efforts, and enhance their ability to handle a high volume of incidents, ultimately bolstering overall cybersecurity defenses.
4. Malware Detection and Prevention
Another area where AI can help in cybersecurity is malware detection and prevention. AI-powered systems leverage machine learning algorithms to analyze the characteristics and behaviors of known malware and identify patterns that can indicate the presence of new and emerging threats.
These systems can detect and classify malware based on file signatures, code analysis, behavioral patterns, and network traffic anomalies. Through continuous learning, AI can adapt to evolving malware techniques and enhance its detection capabilities.
Additionally, AI assists in proactive prevention by identifying vulnerabilities, analyzing system logs for suspicious activities, and providing real-time threat intelligence, enabling organizations to fortify their defenses and mitigate the risk of malware infections.
5. Security Risk Assessment:
AI plays a significant role in security risk assessment by augmenting traditional methodologies with advanced analytics and automation. By leveraging machine learning algorithms, AI can analyze vast amounts of data from diverse sources, including historical incidents, network logs, user behavior, and threat intelligence feeds.
This analysis enables AI-powered systems to identify patterns, correlations, and potential vulnerabilities that may pose security risks. AI can also automate the assessment process by continuously monitoring systems, detecting anomalies, and generating risk scores in real-time. By combining data-driven insights with human expertise, AI facilitates more accurate and efficient risk assessments, empowering organizations to prioritize and allocate resources effectively to mitigate potential security threats.
Challenges & Limitations of Implementing AI in Cybersecurity
Implementing AI in cybersecurity poses several challenges that organizations must address to ensure effective and secure deployment.
Here are the seven main challenges:
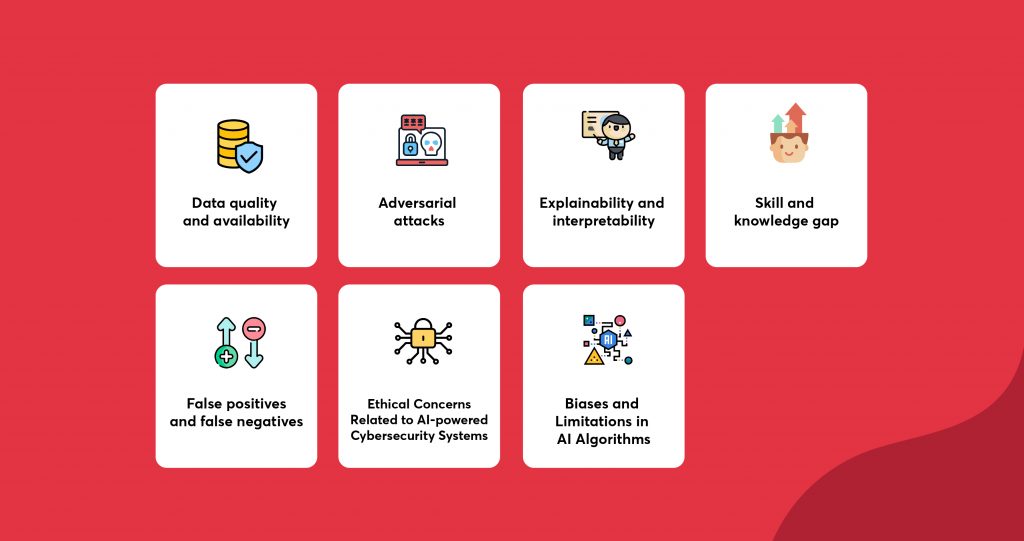
1. Data quality and availability:
AI models heavily rely on high-quality and diverse datasets for training and validation. Obtaining clean, labeled, and representative cybersecurity data can be challenging due to data privacy concerns, data scarcity, and the rapidly evolving threat landscape.
2. Adversarial attacks
Adversaries can exploit vulnerabilities in AI systems, such as poisoning training data or manipulating inputs, to deceive or evade detection. Protecting AI models against adversarial attacks requires robust defenses and continuous monitoring to identify and mitigate potential vulnerabilities, which might again add to the cost.
3. Explainability and interpretability
AI algorithms often operate as black boxes, making it challenging to understand how they arrive at specific decisions or predictions. In cybersecurity, explainability is crucial for understanding the rationale behind threat detections, addressing compliance requirements, and gaining the trust of stakeholders.
4. Skill and knowledge gap
Implementing AI in cybersecurity requires expertise in both fields. Organizations face the challenge of acquiring and retaining professionals with a deep understanding of AI and cybersecurity, as well as interdisciplinary skills to effectively develop, deploy, and maintain AI-powered security systems.
5. False positives and false negatives
AI systems may produce false positives or false negatives. Balancing the detection accuracy to minimize false positives and false negatives is a challenge that requires continuous monitoring, refinement of models, and fine-tuning of thresholds to ensure optimal performance.
6. Ethical Concerns Related to AI-powered Cybersecurity Systems:
While AI offers immense potential in enhancing cybersecurity, it also raises ethical concerns that must be carefully addressed. One key concern is the potential invasion of privacy. AI systems collect and analyze vast amounts of data, including sensitive user information. It is crucial to ensure that AI-powered cybersecurity systems adhere to strict privacy regulations and handle data in a responsible and transparent manner.
7. Biases and Limitations in AI Algorithms:
AI algorithms are only as effective as the data they are trained on. If the training data is biased or incomplete, the AI system may generate biased or inaccurate results. That can lead to false positives or false negatives in threat detection, potentially undermining the effectiveness of cybersecurity defenses. It is vital to ensure that AI algorithms are trained on diverse and representative datasets to minimize biases and improve accuracy.
Emerging Trends: The Future of AI in Cybersecurity
The field of cybersecurity is in a perpetual race against evolving threats, requiring innovative solutions to stay ahead of cybercriminals. AI has emerged as a game-changer in this domain, offering immense potential to bolster defenses and counter sophisticated attacks.
As AI continues to advance, new and exciting trends are reshaping the landscape of cybersecurity. From deep learning and explainable AI to the fusion of AI with edge computing and IoT security, these trends are poised to shape the future of cybersecurity, empowering organizations to proactively protect their assets and adapt to the ever-changing threat landscape.
Deep Learning and Neural Networks
Deep learning, a subset of AI, is gaining momentum in the world of cybersecurity. Today, deep neural networks are being used to analyze complex patterns and features in data, enabling more accurate threat detection and prediction. The use of deep learning models has enhanced anomaly detection, malware analysis, and the identification of advanced persistent threats (APTs).
Generative AI for Adversarial Defense
Adversarial attacks involve manipulating AI systems by injecting malicious inputs. Generative AI techniques, such as Generative Adversarial Networks (GANs), are now used to create robust defenses against adversarial attacks. By generating synthetic adversarial samples, AI systems can be trained to recognize and defend against previously unseen attack vectors.
Natural Language Processing (NLP) for Threat Intelligence
NLP techniques are leveraged to analyze vast amounts of unstructured text data, such as security reports, blogs, and social media posts, to extract actionable threat intelligence. AI-powered NLP models can assist in understanding and categorizing cybersecurity-related information, identifying emerging threats, and facilitating proactive defense measures.
AI-powered Security Orchestration, Automation, and Response (SOAR)
SOAR platforms leverage AI to streamline and automate incident response processes. AI algorithms can analyze security alerts, prioritize incidents, and trigger predefined response actions, reducing response times and minimizing manual efforts. The integration of AI into SOAR empowers security teams to efficiently manage and respond to a high volume of security incidents.
In conclusion, the integration of AI in the field of cybersecurity has proven to be a game-changer. AI offers advanced capabilities in detecting and responding to cyber threats, automating routine tasks, and providing valuable threat intelligence. Its ability to analyze vast amounts of data, identify patterns, and adapt to new and evolving threats empowers organizations to stay ahead of cybercriminals and protect their critical assets.
Looking ahead, the future potential of AI in cybersecurity is promising. As AI technology continues to evolve, new trends such as adversarial AI and explainable AI will shape the landscape of cybersecurity. However, it is crucial to approach AI integration with caution, considering potential ethical concerns, biases, and limitations.
A holistic approach to cybersecurity is essential, where AI complements the expertise of cybersecurity professionals rather than replacing them. The collaboration between human intelligence and AI-powered systems is crucial in effectively combating cyber threats. Cybersecurity professionals must continuously update their skills and knowledge to adapt to the changing AI-driven landscape.